In today’s rapidly evolving digital landscape, the integration of AI for business analytics is fundamentally transforming how organizations harness data for strategic decision-making. As we approach 2024, businesses increasingly recognize the necessity of data-driven insights to remain competitive and innovative. This comprehensive blog will delve into the latest trends, technologies, and strategies surrounding AI for business analytics, illustrating how companies can unlock new dimensions of data intelligence to enhance operations, improve customer experience, and drive growth.
Key Takeaways
- AI Transformation
- Real-Time Insights
- Natural Language Processing (NLP)
- Automated Machine Learning (AutoML)
- IoT Integration
- Data Quality Matters
- Cultivating a Data-Driven Culture
- Challenges Ahead
- Future Trend
- Enhanced Customer Experience
Table of Contents
1. The Evolution of AI in Business Analytics
The journey of AI in business analytics has undergone a significant transformation over the past decade. Initially, organizations relied on basic analytics tools that merely interpreted historical data, offering insights into past performance without the capability for predictive analysis. However, with advancements in machine learning (ML) and deep learning, AI has elevated business analytics into a proactive discipline that not only analyzes past data but also forecasts future trends.
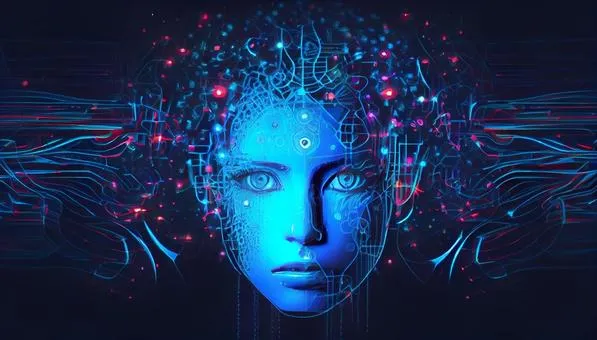
Key Benefits of AI for Business Analytics:
- Enhanced Predictive Analytics: By employing sophisticated algorithms capable of analyzing vast datasets, businesses can forecast trends and customer behaviors more accurately than ever before. For example, retailers can anticipate consumer buying patterns, allowing them to optimize inventory management and reduce waste.
- Real-Time Insights: AI-driven analytics tools can process data in real-time, enabling businesses to respond swiftly to market fluctuations and customer needs. This agility can be the difference between capitalizing on an emerging opportunity and falling behind competitors.
- Automated Reporting: Routine analytics tasks, such as generating reports, can be automated with AI, freeing up valuable human resources for more strategic initiatives. This shift allows employees to focus on high-value activities that drive business growth.
2. Major Trends in AI for Business Analytics in 2024
a. Increased Use of Natural Language Processing (NLP)
Natural Language Processing (NLP) is a branch of AI that allows machines to understand and interpret human language. As we enter 2024, businesses will increasingly leverage NLP within their analytics platforms, allowing non-technical users to extract insights through conversational interfaces. This democratization of data analysis means that employees across all levels can engage with data without needing extensive technical expertise.
Applications of NLP in Business Analytics:
- Voice-Activated Data Queries: Employees can ask questions about their data using voice commands, making it easier to access insights.
- Sentiment Analysis: NLP can be used to gauge customer sentiment from social media and customer feedback, providing valuable insights into brand perception.
b. Automated Machine Learning (AutoML)
Automated Machine Learning (AutoML) represents a significant advancement in making AI more accessible to businesses. By automating model selection and tuning processes, AutoML simplifies the implementation of predictive analytics. In 2024, more businesses will adopt AutoML solutions, allowing them to effectively and efficiently leverage AI for business analytics.
Benefits of AutoML:
- Efficiency: AutoML reduces the time and effort required to build machine learning models, enabling organizations to deploy solutions faster.
- Accessibility: Non-experts can utilize AutoML tools to derive insights from data without needing a deep understanding of data science.
c. Integration with Internet of Things (IoT)
The proliferation of IoT devices generates massive amounts of data, which can yield valuable insights when analyzed correctly. In 2024, AI for business analytics will increasingly integrate with IoT systems, allowing businesses to analyze real-time data streams. This integration provides a comprehensive view of operations and customer interactions, facilitating smarter decision-making across industries such as manufacturing, logistics, and retail.
Use Cases of IoT and AI Integration:
- Predictive Maintenance: In manufacturing, AI analyzes data from IoT sensors on machinery to predict maintenance needs, reducing downtime and costs.
- Smart Inventory Management: Retailers can use IoT devices to monitor stock levels in real-time, enabling automated restocking processes based on customer demand.
d. Enhanced Data Visualization Techniques
As data complexity increases, so does the need for effective visualization tools. In 2024, businesses will leverage advanced data visualization techniques powered by AI to present insights clearly and intuitively. Enhanced dashboards will allow stakeholders to interact with data dynamically, promoting a deeper understanding of trends and metrics.
Examples of Advanced Visualization Techniques:
- Interactive Dashboards: Users can customize views and drill down into specific data points, fostering a more engaging analysis experience.
- Storytelling with Data: AI can help narrate data insights through visualizations, guiding users through key findings and actionable recommendations.
3. Strategies for Implementing AI for Business Analytics
a. Establish Clear Objectives
Before adopting AI for business analytics, organizations must define clear objectives aligned with their overall business goals. Whether aiming to improve customer engagement, optimize supply chains, or enhance marketing strategies, having a defined purpose will guide the selection of the right tools and technologies.
Steps to Define Objectives:
- Identify Key Performance Indicators (KPIs): Determine what metrics are crucial for measuring success in relation to business goals.
- Conduct Stakeholder Interviews: Engage with various stakeholders to understand their analytics needs and expectations.
b. Invest in Quality Data
Data quality is paramount when implementing AI for business analytics. Organizations should ensure that their data is accurate, complete, and timely. Establishing robust data governance frameworks will help maintain data integrity and facilitate better decision-making.
Best Practices for Ensuring Data Quality:
- Data Cleansing: Regularly clean and validate datasets to eliminate inaccuracies and inconsistencies.
- Data Integration: Use ETL (Extract, Transform, Load) processes to integrate data from disparate sources, ensuring a comprehensive view.
c. Foster a Data-Driven Culture
For AI for business analytics to be successful, organizations must cultivate a data-driven culture. This involves training employees to understand and leverage data insights in their day-to-day activities. Encouraging collaboration between data teams and business units will promote a shared understanding of data’s value.
Strategies to Foster Data-Driven Culture:
- Training Programs: Offer training sessions on data analytics tools and methodologies to empower employees.
- Data Accessibility: Provide easy access to data and analytics tools for all employees, encouraging them to utilize data in their roles.
d. Choose the Right Technology Partners
Selecting the right technology partners is crucial for successful AI implementation. Organizations should evaluate vendors based on their expertise in AI for business analytics, the scalability of their solutions, and their commitment to customer support. Collaborating with experienced partners will ensure a smoother transition to AI-driven analytics.
Key Criteria for Evaluating Vendors:
- Reputation and Experience: Look for vendors with a proven track record in AI and analytics.
- Customer Support: Assess the level of customer support and training offered by potential partners.
To explore more, click here
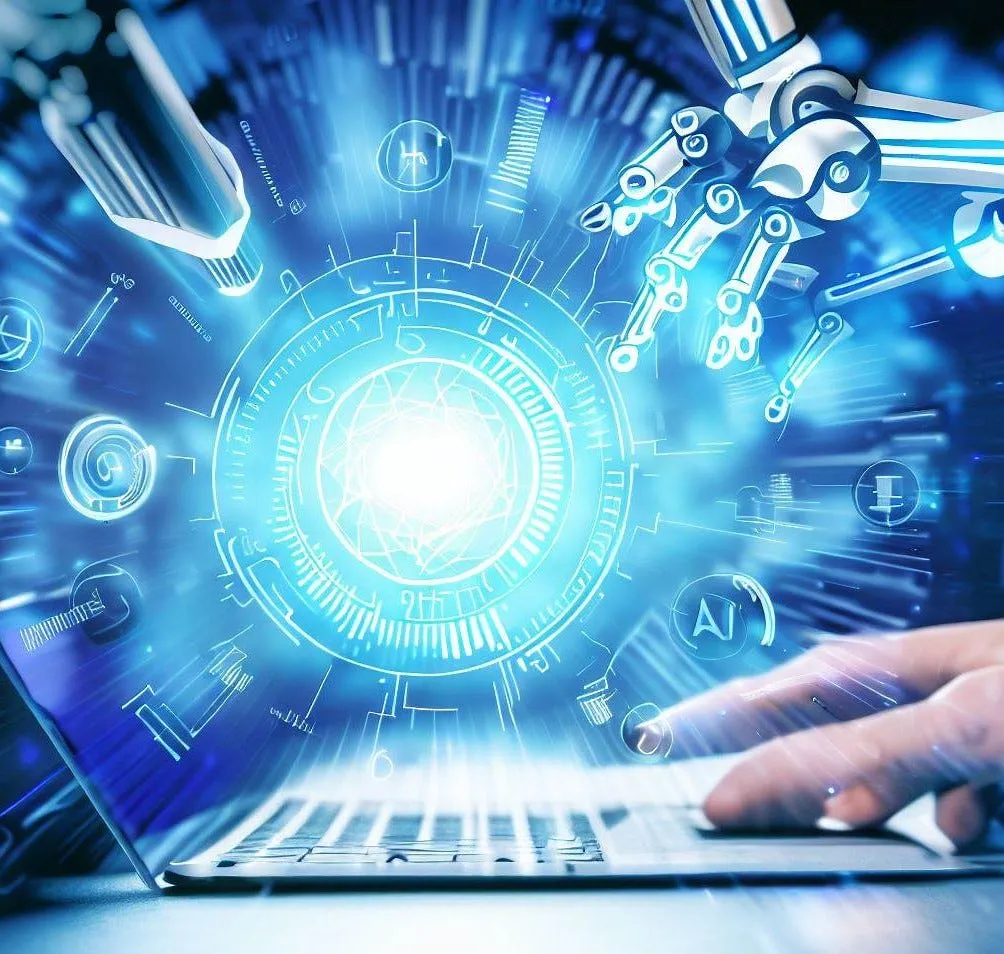
4. Challenges in Adopting AI for Business Analytics
a. Data Privacy and Security Concerns
As businesses harness AI for business analytics, they must navigate data privacy and security issues. With stricter regulations, such as GDPR, organizations must ensure compliance while managing customer data. Implementing robust security measures will be essential to protect sensitive information.
Strategies for Addressing Data Privacy:
- Data Anonymization: Use techniques to anonymize personal data, reducing the risk of exposure.
- Regular Audits: Conduct regular audits of data practices to ensure compliance with privacy regulations.
b. Skill Gaps in the Workforce
While AI tools simplify analytics processes, there is still a need for skilled professionals who can interpret data and derive actionable insights. Organizations may face challenges in bridging skill gaps within their workforce. Investing in training and development programs will be critical to empower employees with the necessary skills.
Approaches to Address Skill Gaps:
- Upskilling Initiatives: Create training programs focused on analytics and data interpretation for current employees.
- Collaboration with Educational Institutions: Partner with universities and colleges to develop programs that produce skilled graduates in data analytics.
c. Resistance to Change
Change management is another hurdle organizations must address. Employees may resist adopting AI-driven tools due to fear of job displacement or unfamiliarity with new technologies. It’s important for leadership to communicate the benefits of AI for business analytics and involve employees in the transition process.
Tactics to Mitigate Resistance:
- Transparent Communication: Clearly communicate the benefits of AI adoption and how it enhances rather than replaces jobs.
- Involvement in Implementation: Involve employees in the implementation process to foster a sense of ownership and reduce resistance.
5. Future Outlook for AI in Business Analytics
The future of AI for business analytics is bright, with continued advancements expected in the coming years. As AI technologies mature, organizations will gain access to even more sophisticated tools that provide deeper insights and predictive capabilities. In particular, the integration of AI with quantum computing holds promise for processing vast datasets at unprecedented speeds, revolutionizing how businesses approach analytics.
The Role of AI in Enhancing Customer Experience
One of the most significant impacts of AI for business analytics will be in enhancing customer experience. By analyzing customer data, businesses can tailor offerings to individual preferences, anticipate needs, and provide personalized recommendations. This level of customization will become increasingly important as consumer expectations continue to rise.
Examples of Enhanced Customer Experiences:
- Personalized Marketing: AI can analyze customer behavior to deliver targeted marketing campaigns, improving engagement and conversion rates.
- Proactive Customer Service: Predictive analytics can anticipate customer issues, allowing businesses to address problems before they escalate.
The Impact on Decision-Making Processes
AI for business analytics will also transform decision-making processes. Organizations will shift from reactive to proactive strategies, utilizing predictive analytics to inform decisions before issues arise. This shift will empower businesses to adapt to market changes swiftly and maintain a competitive edge.
Benefits of Proactive Decision-Making:
- Reduced Risk: By anticipating challenges, businesses can implement strategies to mitigate risks
and avoid costly mistakes. This proactive approach not only enhances agility but also fosters a culture of continuous improvement within organizations.
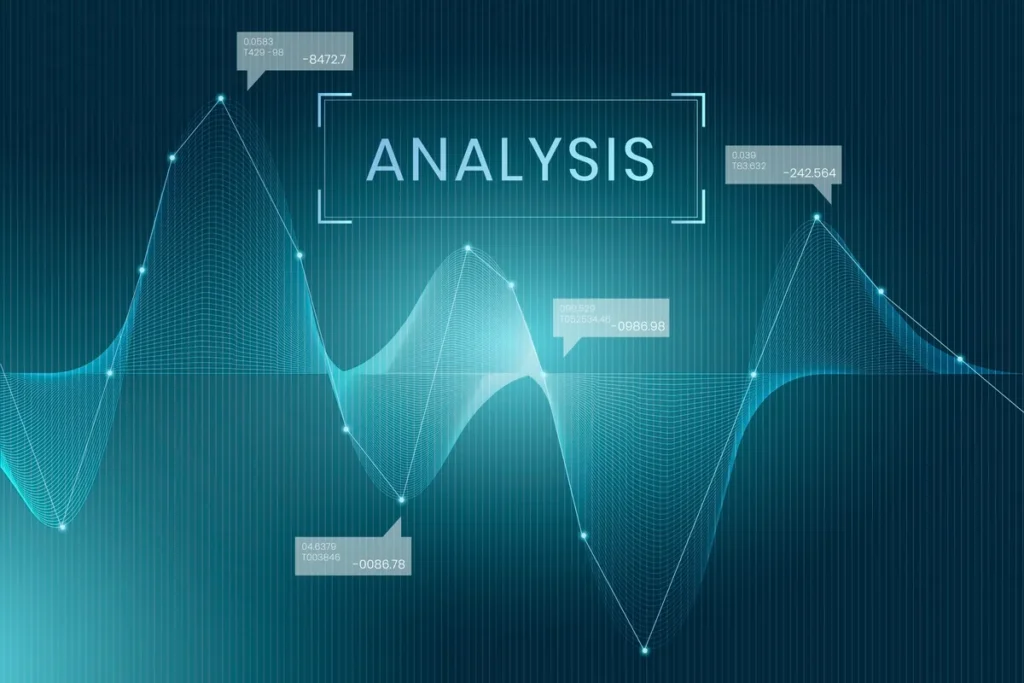
F&Q
1. What is AI for business analytics?
AI for business analytics refers to the application of artificial intelligence technologies to analyze data and provide actionable insights. This includes using machine learning algorithms, natural language processing, and automated reporting tools to enhance decision-making processes.
2. How does AI improve predictive analytics?
AI enhances predictive analytics by utilizing advanced algorithms that analyze historical data to identify patterns and forecast future trends. This capability allows businesses to anticipate customer behavior and market changes effectively
3. What role does natural language processing (NLP) play in business analytics?
NLP enables users to interact with analytics tools using everyday language, making data analysis more accessible to non-technical users. This allows broader participation in data-driven decision-making across an organization.
4. What are the benefits of using automated machine learning (AutoML)?
AutoML simplifies the process of building machine learning models by automating tasks such as model selection and tuning. This accessibility allows organizations to leverage AI for analytics without needing extensive data science expertise.
5. How can businesses ensure data quality when implementing AI?
Businesses can ensure data quality by establishing robust data governance frameworks, regularly cleaning and validating datasets, and integrating data from various sources to create a comprehensive view.
6. What challenges might organizations face when adopting AI for business analytics?
Organizations may encounter challenges such as data privacy and security concerns, skill gaps in the workforce, and resistance to change from employees. Addressing these challenges is critical for successful AI implementation.
7. How will AI impact customer experience in the future?
AI is expected to significantly enhance customer experience by providing personalized recommendations and proactive customer service, allowing businesses to meet individual customer needs more effectively
8. What is the future outlook for AI in business analytics?
The future of AI in business analytics is promising, with expected advancements such as quantum computing and deeper integration with IoT, which will revolutionize data processing and analytical capabilities.
9. How can organizations foster a data-driven culture?
Organizations can foster a data-driven culture by providing training programs, ensuring easy access to data, and promoting collaboration between data teams and other business units.
10. What technologies should businesses consider for implementing AI in analytics?
Businesses should evaluate technologies that offer advanced analytics capabilities, user-friendly interfaces, strong customer support, and integration options with existing systems
Conclusion
As we look ahead to 2024, AI for business analytics will continue to unlock new dimensions of data intelligence, enabling organizations to make informed decisions, enhance customer experiences, and drive growth. By embracing the latest trends, implementing effective strategies, and addressing challenges head-on, businesses can harness the full potential of AI-driven analytics.
This comprehensive exploration of AI for business analytics highlights the transformative power of these technologies and the strategies organizations can adopt to stay ahead. By understanding the trends and preparing for the future, businesses can maximize their data intelligence and achieve long-term success in an increasingly data-driven world.
Visit our Website today to learn more : Wodo Digital.